ABSTRACT
This paper describes a novel strategy to Radio-Frequency Identification (RFID) tag detection for human–robot interaction (HRI) purposes. The anisotropic detection pattern of the RFID reader antenna is combined with a probabilistic algorithm to obtain a coarse angular position relative to the RFID reader that can be used, for example, for behavioral control based on proxemics areas around the robot. The success rate achieved is suitable for HRI purposes. The paper presents experimental results on a detection model for the reader.
RFID RELATED WORK AND SOCIAL ROBOTICS CONSTRAINTS
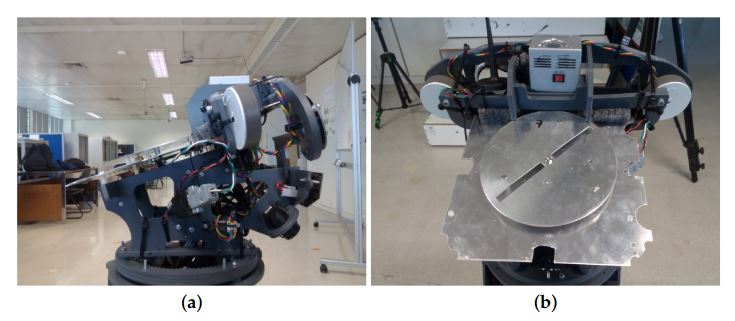
Figure 1. Radio-Frequency Identification (RFID) antenna attached to the head frame of the MOnarCH robot
The whole software is wrapped as an ROS (Robot Operating System) node so that it can be accessed by anyone registered with the ROS master. The reader only supplies the identification of the tags detected. No RSSI is available. The reader is installed onboard a MOnarCH robot inside the head (see Figure 1).
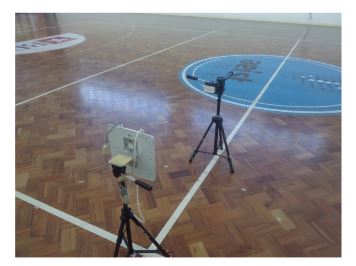
Figure 3. Wide indoors open area used to establish a baseline model
The aforementioned (uncontrolled) factors suggest that the reader can be modeled using a probabilistic-like technique by which to each point in a neighborhood of the reader is assigned a probability of a tag being detected in case it is located at that point. This probability is estimated simply as the percentage of true positive detections. Clearly, this estimate will depend heavily on the environment conditions. The model of the reader was obtained in an open indoors environment (see Figure 3) with a large hemispherical free volume centered at the antenna.
EXPERIMENTS
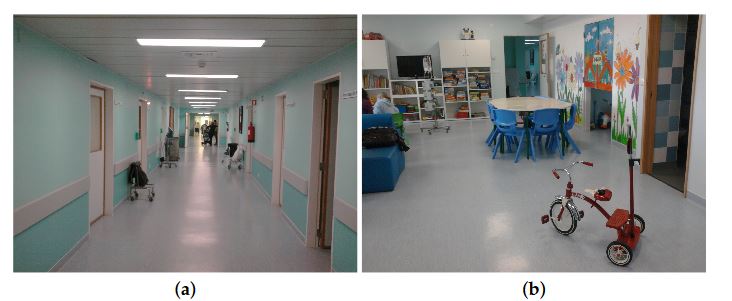
Figure 8. The Instituto Português de Oncologia de Lisboa (IPOLFG) hospital environment. (a) main corridor; (b) playroom
Two views of the main area where the experiments took place at a hospital environment are shown in Figure 8. Side walls are concrete, doors are from wood, the floor is concrete with a plastic cover, and the ceiling is metallic. This environment has similarities with the in-lab test environment, except for the people. The robot wanders through this environment interacting with people and using RFID tag detection to take HRI related decisions.
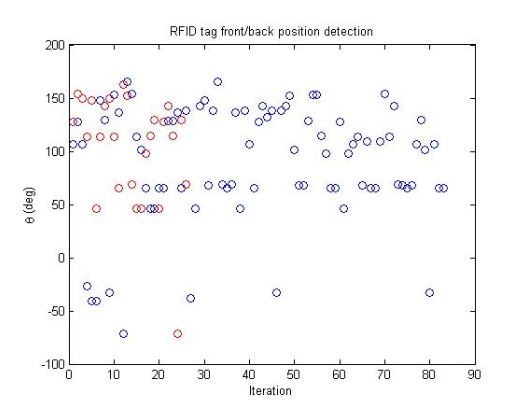
Figure 12. Front/back detection of tags passing by the robot
Figure 12 shows the angular detection when the tag passes by the robot (with the onboard reader) in the corridor shown in Figure 8a. The frontal area of the robot corresponds to angles in the range [0◦, 180◦]. The red/blue marks correspond to the person carrying the tag approaching the robot, head-to-head, passing through the right/left hand side of the robot, respectively, and continuing along the corridor. The plot contains multiple runs.
CONCLUSIONS
The experiments demonstrate a number of features of interest of RFID technology for HRI purposes, in particular those related to proxemics. In ideal conditions, namely without disturbances, the anysotropic detection pattern of the antenna can be used to obtain a rough estimate of the angular position of the tag in the robot frame. The specific antenna pattern considered yield a good agreement for tags in quadrants 2 and 4.
This, in turn, is a clear indicator that good discrimination in all four quadrants can be achieved by combining two (or more) antennas such that the respective detection patterns are adequately oriented (or using a single orientable antenna). For applications requiring rougher angular discrimination, e.g., front/back, a single antenna can yield an interesting performance. The experiments show that environment conditions may significantly influence the performance of the model.
Thus, future work includes (i) identifying in real time the model of the reader installed onboard the robot; and (ii) studying motion strategies for the robot equivalent to a directional antenna that do not disturb the HRI. In future work, dynamic maps containing the localization of people in the neighborhood of the robot, produced using the technique in the paper, can also be merged with dynamic maps produced using other sensors. In the case of the MOnarCH robot, such sensors can be the RGB-D cameras at the head and the lasers at the bottom of the robot.
Source: University De Lisboa
Authors: Joao S. Sequeira | Duarte Gameiro